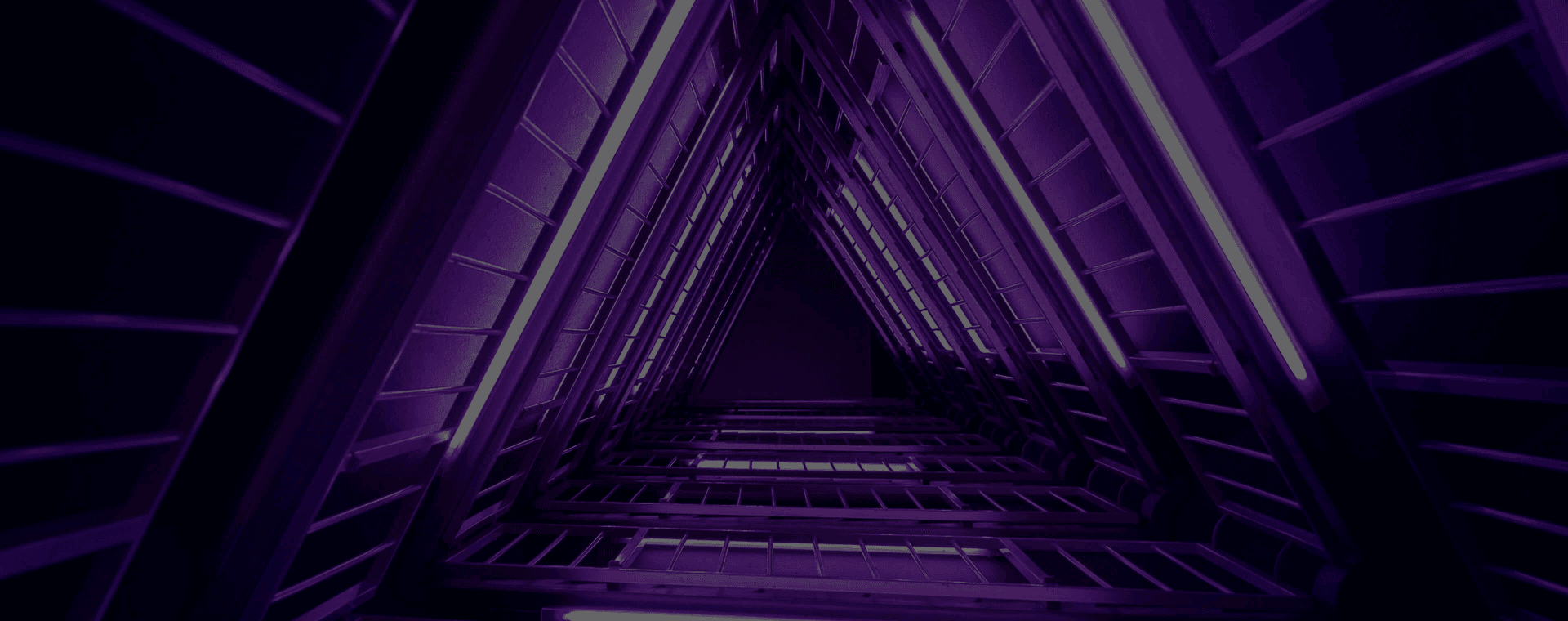
Video Analytics Trends Part 4: Cloud and Edge Processing

Graeme Woods
Global Business Analyst
This is part four of a five-part series about trends in video analytics and is about cloud and edge processing.
Last time, I discussed composite detections, where the analytics combines multiple detections to provide a more valuable and accurate detection. In previous articles, I’ve discussed combining structured and unstructured data and how AI is going to help provide more insightful reporting from video analytics.
In video analytics, the process includes:
● video decoding (converting it from a highly compressed format into frames for analysis);
● video analysis (using multiple machine learning models that are combined into an analytic and managing alerts);
● data storage (detections and historical video).
Cloud and edge processing is a change in the way that video data is processed. Traditionally, video analytics relied on enterprise servers with 1, 2, 4 or more high-end Nvidia GPUs to decode and manage video and run the analytics, which include deep learning models. This is called on-premises processing.
The main drawback of on-premises installations is the cost of the server hardware and maintenance costs. An enterprise server with four GPUs may cost tens of thousands of dollars and have an effective life of only three to five years. Servers need regular patching to protect against vulnerabilities. There may be a significant lead time and difficulties in getting the correct hardware configuration. Vital data needs to be backed up or mirrored offsite.
The server will also need to be kept in a special server room (they are too noisy to keep in the office), adding more costs, and power consumption can be substantial. Twenty enterprise servers with multiple GPUs and their required air-conditioning can cost over $10,000 per month in electricity charges.
In contrast, edge devices have low power consumption with no moving parts and are rugged enough to be placed almost anywhere. They are passively cooled, don’t need regular patching and have a small fraction of the capital costs of an enterprise server. They are not as powerful as an on-premises server but offer an attractive cost/benefit ratio.
Cloud services include public clouds such as AWS, Azure or Google Cloud or private clouds offered by vendors. Cloud servers have no capital costs at all. The only cost is operational.
There are two ways to use a cloud server. Firstly, you can run a cloud server that is similar to an on-premises server. This still requires patching, but there is no hardware purchasing or maintenance. On premises servers do not optimize costs because the server is either on or off – there is little opportunity to scale costs with load.
The second, preferred cloud model is serverless, where the solution combines a series of predefined services. This uses cloud resources efficiently because there is only a charge when a service is being used.
Cloud services for video analytics offer an easy way to federate sites (monitor multiple physical locations), and there is no software to maintain. It is easy for the vendor to include software upgrades and new analytics. When there is a peak period, it is easy to scale up capacity, and then reduce it when it is quiet.
Cloud services are becoming practical with the increasing use of H.265 video compression, which reduces bandwidth. There are many defined services that allow management and storage of video and deployment of machine learning models.
I expect that edge devices and the cloud will increasingly be used together in a hybrid cloud model. The following diagram shows this in operation:
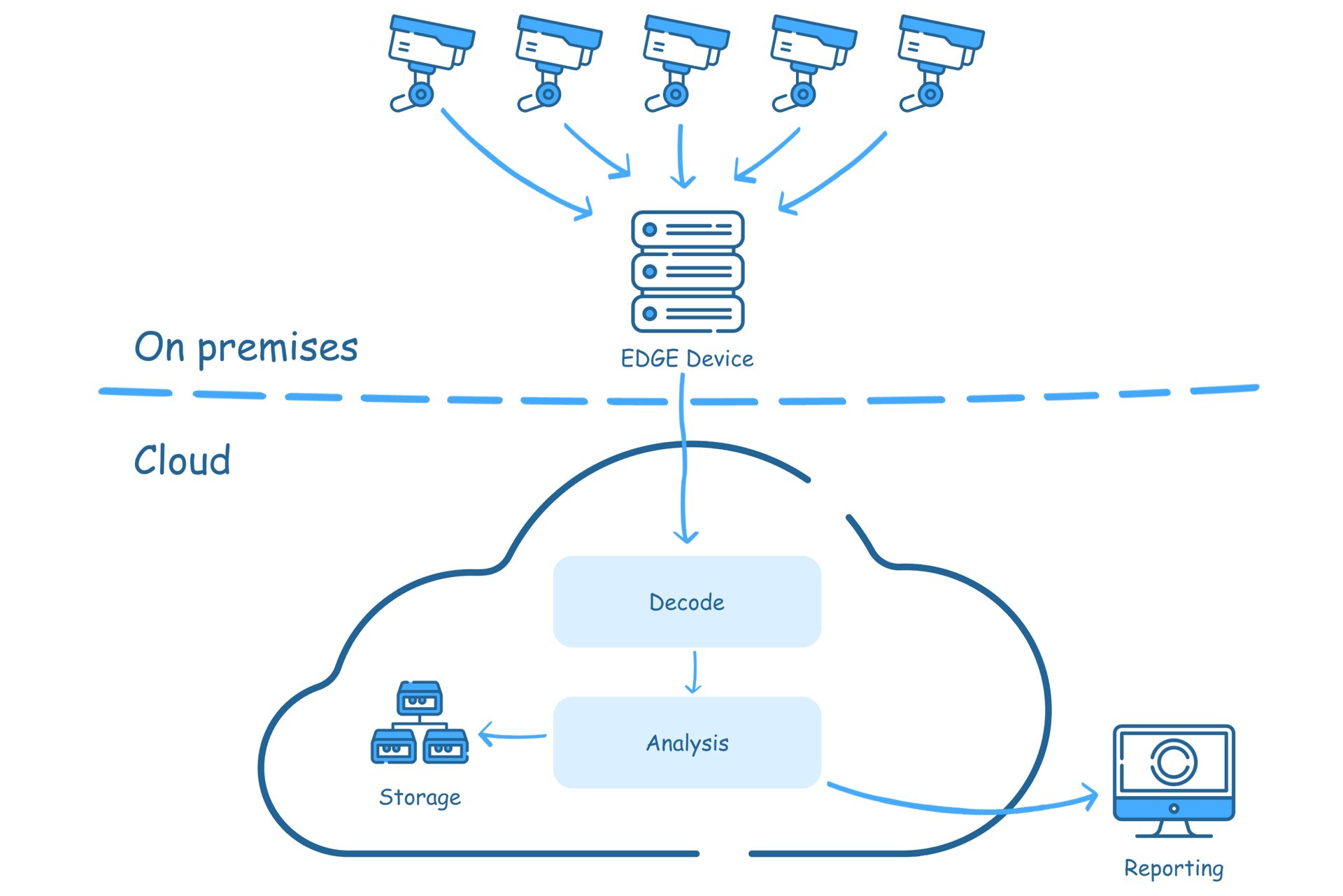
Edge devices will perform some initial processing to determine whether an event of interest has occurred. This could be something out of the ordinary, or a specific analytic. The edge device would act as a filter to reduce the volume of data sent to the cloud. In this case, we would trade off a high positive rate for a very low false negative rate.
On the cloud, a serverless solution would decode compressed video then process the video against several algorithms to develop a composite detection. The operation of the solution would be triggered by incoming video frames to process. This solution would then store video in a cloud object store and write results to a serverless database table.
Scylla Asteria™ edge monitoring device performs accurate physical threat detection and advanced analysis on edge, running effective gun detection, smoke & fire detection, industrial temperature monitoring, perimeter intrusion detection, and aggressive behavior detection, among others. Scylla’s software filters out up to 99% of non-actionable events so that the users can see only relevant alerts.
I believe that the hybrid cloud combination of an edge device and the cloud will rapidly replace on-premises servers except for rare situations where the customer doesn’t trust encryption or where there is no connectivity to the cloud. The hybrid cloud model offers more scalability, lower costs and more powerful analytics, as well as easier federated reporting.
More Video Analytics Trends:
Part 1: Combining Structured and Unstructured Data
Part 4: Cloud and Edge Processing
Stay up to date with all of new stories
Scylla Technologies Inc needs the contact information you provide to us to contact you about our products and services. You may unsubscribe from these communications at any time. For information on how to unsubscribe, as well as our privacy practices and commitment to protecting your privacy, please review our Privacy Policy.
Related materials
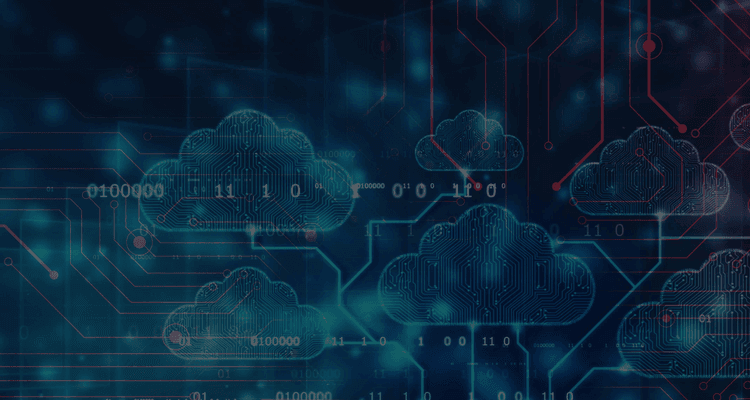
The Transition of Physical Security to the Cloud
The migration to the cloud is one of the latest trends for physical security. Learn what advantages cloud-based technologies have and see how organizations benefit from transition to the cloud.
Read more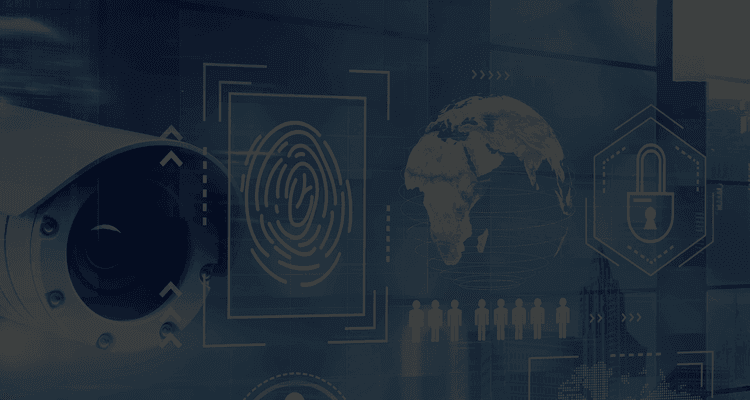
Top Physical Security Trends for 2022
Learn about the top six physical security trends that companies should take into account while rethinking their physical security strategy in 2022.
Read more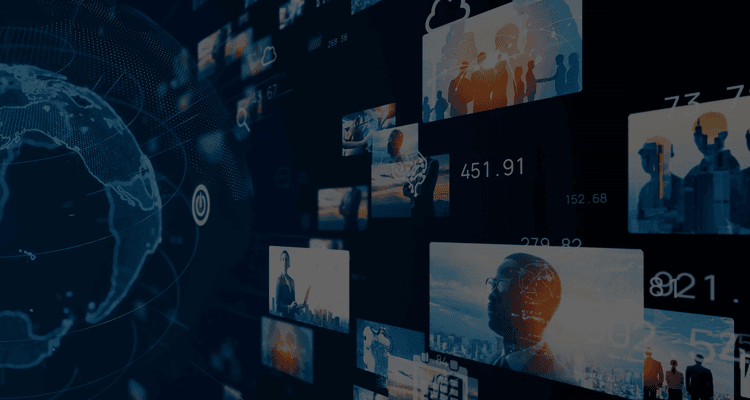
Edge AI Solutions for Physical Security
Edge AI technology is a cost-effective, reliable, scalable and easy-to-use solution. Learn how edge AI solutions can be effectively used for physical security.
Read more